Title
TelMed5G – Professional telemedical platform for 5G networks
Organization
medVC.eu sp. z o.o. https://medvc.eu/
Experiment description
Currently, the type and level of medical competence is highly location-dependent. The procedures involving travel of either the patient or medical personnel are risky, costly and time-consuming. Especially in emergency situations time constraints often make multidisciplinary expertise impossible. Remote collaboration of doctors is an approach to solving these issues, saving both lives and money.
medVC is a scalable telepresence platform designed to be deployed in hospitals to enable real-time audio-video communication and remote collaboration between doctors. medVC consists of hardware medical end-points and extended services placed in the cloud. We focus on achieving the highest possible quality of medical picture and sending multiple HD video streams coming from cameras, microscopes, endoscopes, surgical robots and other medical equipment. We found out from the medical professionals we cooperate with that pure audio-video communication is not enough. Therefore, medVC is enriched with extended collaboration services taking advantage of the cloud architecture.
medVC is controlled via an intuitive, smartphone-like user interface using a touch-screen display. medVC gives all participants the possibility to pause videos, draw on the picture, take snapshots, collaboratively work on DICOM images, record a session or even broadcast it live over the Internet. With a dedicated medical video portal, medVC allows doctors to publish recordings of their surgeries with just one click. Furthermore, medVC can transmit stereoscopic (3D) video from surgical robots or 3D endoscopes. All these features make medVC a superb remote collaboration and education tool for medicine.
medVC user interface
As medVC is already used by hospitals in Austria, France, Germany, Italy, Luxembourg, the Netherlands, Poland and Spain, which often have the need to conduct medical collaborative calls between each other, our company provides a reliable and scalable solution that allows to hold multipoint calls. The medVC Multi-point Control Unit (MCU), in opposition to standard videoconferencing MCUs, is an intelligent packet reflector, replicating audio-video packets and sending them to the appropriate receivers in order not to recode medical video and to provide maximum quality, needed for medical applications. Furthermore, the version of the medVC MCU used in this experiment provides dynamically programmable and agile routing of data flows. Based on the current participants of a remote medical collaboration session and their locations it replicates data packets only to the recipients that are closest to it. This allowed to deploy the medVC MCU as a cloud solution, intelligently routing traffic between MCU instances and end-points. The diagram below illustrates how the intelligent routing allows to avoid sending all streams between endpoints and MCUs, saving the necessary bandwidth.
The medVC MCU and routing
The introduction of edge processing within 5G networks seemed to be a perfect solution for deploying the medVC MCU on the edge nodes. Such a topology should help save network bandwidth, as video streams don’t have to be sent to the core cloud, but can be routed in the edge cloud.
A second interesting aspect of processing on edge nodes is the possibility of dynamic recoding of video streams. As medVC focuses on very high quality images, the bandwidth we use is high, about 8 Mbps for a Full HD stream and in some scenarios 20 Mbps for 4K streams. Moreover, since medVC is capable of sending and receiving multiple videos, the bandwidth usage in multiuser scenarios grows quickly. Furthermore, video recording functions provided by medVC allow doctors to store videos with higher bitrate than the one used in a live telemedical session, which also adds to the bandwidth usage and additionally requires video streams in two different qualities to be encoded simultaneously on each medVC terminal. With the introduction of edge processing, we should be able to encode only one high-bitrate stream on the terminals and recode it on the edge node for the live session. Furthermore, medVC also allows live streaming for mobile devices, that usually have lower computational power and network capacity, which limits the possible bitrate of the incoming video. The introduction of video recoding on edge nodes for recipients of medVC streaming should both save bandwidth and lower the processing power requirement for the terminals.
In our work, we used the eHealth5G facilities of the 5GinFIRE project. eHealth5G provided the network resources we needed, the laboratory space to deploy our devices and the medical equipment that was crucial for acquiring medical-grade video. The diagram below shows the architecture of our experiment.
General architecture of the experiment
In the diagram above, the red lines represent high quality/bitrate video streams sent from the medVC terminals to the edge nodes. The numbers by the lines represent the source terminal of each stream. The medVC MCU and recoding/routing software has been installed on the edge nodes as VNFs. The recoding/routing software has been recoding the high bitrate streams to medium bitrate streams for the telemedical sessions (blue lines) and forwarding them to the MCU on the same edge node. The MCU would then identify which clients were connected to it and which to the other MCU deployed on the other edge node, and distribute the streams accordingly. In the test scenario we used 3 medVC terminals and 2 MCUs as a proof of concept, but the topology is scalable to a higher number of both. The recoding/routing software has also been responsible for forwarding the high quality stream (red lines) to the recording service placed in the core cloud. In the scenario with streaming to a mobile device, the recoding/routing software on the appropriate edge node has also been preparing a lower bandwidth stream and sending it to the mobile device (black line).
The list below shows the results we planned to reach in our experiment:
- Validation of the medVC MCU with a dynamic routing distributed topology in a 5G network, taking advantage of deployment on edge nodes
- Measurement of the bandwidth savings of the distributed medVC MCU topology
- Validation of edge node recoding of high quality video for live telemedical sessions and mobile devices
- Measurement of the bandwidth savings related to the usage of node recoding
- Validation of a prototype of management software for automatic on demand deployment of MCU and recoding/routing software images on edge nodes
Tuning the recoding parameters to achieve adequate quality for medical purposes, involving subjective quality testing with the help of doctors.
Results
Validation of the medVC MCU with a dynamic routing distributed topology in a 5G network, taking advantage of deployment on edge nodes
The MCU was deployed on both edge nodes, according to the diagram above, and also on the core node, in order to receive the signal for the recording service. First, every machine was checked if it was successfully making requests to the DNS service, enabling locating them in the dynamically deployed environment. Subsequently, the DNS was tested whether it was providing up-to-date IP addresses of the machines in the experiment. The next validated issues were related to the main features of our software. The MCU in the core node was picking up incoming calls from medVC terminals correctly and was dynamically assigning the proper MCU on one of the edge nodes. The chosen MCU was observed to be setting up the connections with the medVC terminals correctly. Furthermore, the inter-MCU channel was also verified to be establishing correctly. Therefore, all communication paths were verified working as expected.
Measurement of the bandwidth savings of the distributed medVC MCU topology
In order to measure the bandwidth savings obtained due to the utilization of the distributed medVC MCU topology, two setups were established and experimented on:
- A model of the classic single-MCU setup, with one of the medVC endpoints and the mobile device located closer (in the network link sense) to the MCU and two other medVC endpoints located farther from it (connected to another edge node). The figure below illustrates this setup and lists the bitrates measured in that scenario.
Single MCU setup with average measured bitrates
- The target setup consisting of two medVC MCUs deployed on two edge nodes, with one of the medVC endpoints and the mobile device located closer to one of the MCUs and two other medVC endpoints located closer to the other MCU. The below figure illustrates this setup and lists the bitrates measured in that scenario, as well as the differences in comparison with the above setup.
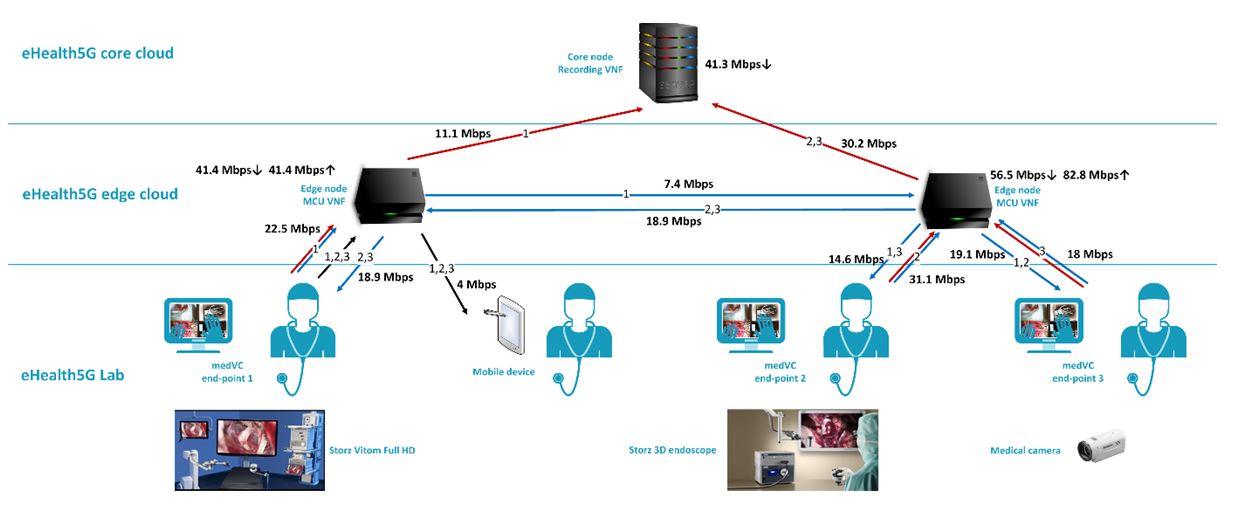
Distributed MCU setup with average measured bitrates
The results described above lead to the following conclusions regarding the effects of using the distributed medVC MCU setup:
- the overall bitrate measured on the link between the MCUs drops significantly
- the overall bitrates of ingoing and outgoing traffic on the MCUs drops significantly
Validation of edge node recoding of high quality video for live telemedical sessions and mobile devices
As described previously, medVC focuses on very high quality images and it is capable of sending and receiving multiple videos, so the bandwidth usage in multiuser scenarios is significant. Furthermore, video recording functions provided by medVC allow doctors to record videos with higher bitrate than the one used in a live telemedical session, which also adds to the bandwidth usage and additionally requires video streams in two different qualities to be encoded on the medVC terminals. medVC also allows live streaming for mobile devices that usually have a lower computational power and network capacity, which limits the possible bitrate of the incoming video. The introduction of video recoding on edge nodes for recipients of medVC streaming should both save bandwidth and lower the processing power requirement for the terminals. The below figure depicts a block scheme of the full processing pipeline for a single video stream, showing the main processing stages.
Flow of a single video stream through the pipeline
The pipeline depicted above was instantiated for each original video stream on both edge nodes. Initially, the solution was tested locally using dedicated set of scripts mocking the incoming RTP stream, the RTP sink of the MCU and the TCP sink of the recording service. Subsequently, the solution was deployed on an edge node where interoperation with MCU was added, since in our experiment the pipeline had to be constructed on-demand during connection setup. After this step was successful, the solution was deemed operational and used in the actual experimentation.
During the deployment phase of the software for the experiment, measurements were done in order to evaluate the necessary computational performance of the VNF machines, in order for them to be able to handle live transcoding without issues. These results influenced parametrizing of the VNF descriptors.
Measurement of the bandwidth savings related to the usage of node recoding
The figures below present the bandwidth usage with and without transcoding. The analysed setup is based on a model architecture of a rather standard recording session involving teleconsultation. The transcoding has been set to the optimal preset (more on that further down). The figures outline average bandwidth savings observed empirically on various network links.
Experiment setup with average measured bitrates – no transcoding
As can be seen in the figures, the introduction of edge node transcoding causes significant bandwidth savings on the links between the terminals and the edge nodes.
Experiment setup with average measured bitrates – with transcoding
Another benefit from the usage of transcoding on the edge nodes is the reduced CPU usage on the terminals themselves. The following tables outline CPU usage reduction on a medVC terminal:
CPU usage reduction with transcoding (for telecollaboration)
Average CPU usage [% of core] | ||
Storz Vitom Full HD | Storz 3D endoscope | |
stream generated locally | 366% | 381% |
stream generated on edge node | 281% | 291% |
relative reduction | 23% | 24% |
CPU usage reduction with transcoding (for streaming)
Average CPU usage [% of core] | ||
Storz Vitom Full HD | Storz 3D endoscope | |
stream generated locally | 347% | 362% |
stream generated on edge node | 278% | 292% |
relative reduction | 20% | 19% |
Validation of a prototype of management software for automatic on demand deployment of MCU and recoding/routing software images on edge nodes
In the experiment we have conducted initial testing of the management software for automatic, on demand deployment of MCU and recoding/routing software. During the establishing of a telemedicine videoconferencing session and/or streaming, the software investigates the geographical location of the participants and then can initiate the requested software components on nodes/servers close to the participants, to reach resource usage optimization shown in the other points of this document. The software design has been prepared in a way, that in the future, when edge processing capabilities will be commercially available, we can easily adapt to APIs of available platforms. This way the software stays generic and we can initiate VNFs on demand on networks of various providers.
Tuning the recoding parameters to achieve adequate quality for medical purposes with the use of subjective quality testing
Transcoding is a process that inherently reduces quality by introducing generational degradation [2] to the content. Therefore, initially we suspected that transcoded streams would require higher bit rates than the ones created in a single step. To evaluate this, we made a full reference comparison of the transcoded streams versus the ones created in one step. The QoE evaluation was done by a medical specialist, a senior surgeon from the Poznań University of Medical Sciences, who has been asked to evaluate the quality degradation. The specialist has been presented with several video fragments with medical content using full-reference technique. The aim of the specialist was to evaluate the quality degradation introduced by an additional step of encoding and decoding of the video. Using the input of the specialist we then intended to establish an optimal, higher bit rate of the final stream, in order for the transcoding not to introduce noticeable degradation.
To our surprise, the specialist was unable to notice the degradation unambiguously. In turn, he confirmed that keeping the final bit rate values unmodified, despite the addition of transcoding, was sufficient to achieve a similar level of QoE.
Conclusions
The general goal of the TelMed5G experiment was to validate the feasibility of using the advantages of edge computing in medVC for enhanced routing and transcoding, as well as to measure the benefits of this approach. The experiment results confirm that the new design of the medVC MCU topology and the introduction of recoding on edge nodes will give the medVC platform the advantages of higher scalability, decreased bandwidth consumption and reduction of hardware requirements for the medVC end-points, which will lower the production costs. This will give our company a competitive edge when 5G networking becomes available in commercial networks, and conducting the TelMed5G experiment is part of our strategy to prepare for this to happen.